TinyML Enabling Low-Power Inferencing, Analytics at the Edge
Original Source Here
By John P. Desmond, AI Trends Editor
Edge computing is booming, with estimates ranging up to $61 billion in value in 2028. While definitions vary, edge computing is about taking compute power out of the data center and bringing it as close as possible to the device where analytics can run.
The devices can be standalone IoT sensors, drones, or autonomous vehicles. “There’s one thing in common. Increasingly, data generated at the edge are used to feed applications powered by machine learning models,” stated George Anadiotis, analyst, engineer and founder of Linked Data Orchestration of Berlin, Germany, working on the intersection of technology, media and data, writing in a recent account in ZDnet.
However, “There’s just one problem: machine learning models were never designed to be deployed at the edge. Not until now, at least. Enter TinyML.”
A fast-growing field of machine learning technologies and applications, tiny machine learning (TinyML) is broadly defined as including hardware, algorithms and software capable of performing on-device sensor data analytics at extremely low power. This enables a variety of always-on use-cases, targeting battery-operated devices.
At the inaugural TinyML EMEA Technical Forum held in June, Anadiotis spoke to several founders of TinyML tech.
Pete Warden Explores TinyML at Google
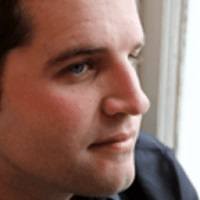
Pete Warden was the founder and CTO of startup Jetpac, which built a product to analyze the pixel data of over 140 million photos from Instagram, and turn them into in-depth guides for more than 5,000 cities around the world. The company was acquired by Google in 2014 and Warren has been a Google Staff Engineer since then. He had fit a machine learning model into a two megabyte footprint, at that time the state of the art in efficiency.
Then he learned some colleagues at Google had a 13 kilobyte machine learning footprint for a system being used to recognize wake words running always on Android devices. That was so the main CPU was not drawing battery power while waiting for the wake word—‘Hey Google’ in this case.
“That really blew my mind, the fact that you could do something actually really useful in that smaller model,” Warden said to Anadiotis. “And it really got me thinking about all the other applications that might be possible if we can run, especially all these new machine learning, deep learning approaches.”
Evgeni Gousev came to the US from Russia more than 25 years ago, intending for it to be a short visit. He is still in the US and is today a senior director at Qualcomm, where he has worked for 16 years. Before that, he was a staff member at IBM’s TJ Watson Research Center, and a professor at Rutgers University. He is a PhD graduate of the Moscow Engineering Physics Institute.
Gousev met Warden in 2018, and learned what was possible using techniques Warden was exploring. Gousev stated, “Peter showed that you can run a deep learning model on 8 bits, without compromising the accuracy much. It was an eye-opening experience for the whole community. It was kind of a mindset shift.”
The two organized a TinyML session on the Google campus, getting strong interest. They set up the TinyML Foundation, and held the first summit in March 2019, seeing active participation by 90 companies.
IoT Growth Fueling Demand for TinyML, Says ABI Research
Market analyst firm ABI recently projected that between 2021 and 2026, the number of IoT connections will reach 23.6 billion, each one representing an opportunity to leverage AI, machine learning and TinyML. The analysts project the TinyML market will grow from 15.2 million shipments in 2020 to 2.5 billion in 2030, according to a press release issued by ABI Research.
The proliferation of AI has fueled the growth of IoT analytics. Data collected from IoT devices is used to train ML models, generating new insights. At the same time, specialized AI chipsets running on edge devices have introduced AI to a range of mobile devices, including automobiles, smart home speakers and wireless cameras.
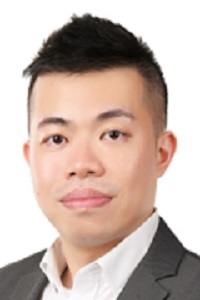
“However, these devices are often too underpowered to make use of all the data flowing across them and struggle to support high-computing performance and high-data throughput, causing latency issues, which is a death knell for AI,” stated Lian Jye Su, AI & ML Principal Analyst at ABI Research.
TinyML enables data analytics to run on low-powered hardware with low processing power and small memory size, assisted by software designed for small-sized inference workloads. This has the potential to enable edge AI to expand beyond traditional markets.
“By bringing AI analytics beyond machine vision, more end users can benefit from smart connected sensors and IoT devices based on sound waves, temperature, pressure, vibration, and other data sources,” Su stated. The benefits of this include data privacy, high interconnectivity, and interaction of various components, and overcoming network bandwidth challenges.
Machine learning is not usually associated with hardware, even though a number of phones and cameras for example, have embedded deep learning models within them. However, embedded AI quickly runs into constraints of power and space, giving TinyML an opportunity, according to an account on the blog of Plug and Play. The company that works to connect startups, corporations, VC firms, universities and government agencies across multiple industries.
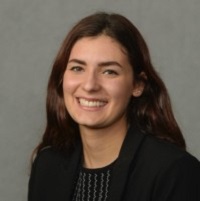
“TinyML brings Machine Learning to the scene by embedding Artificial Intelligence in small pieces of hardware,” stated Margot Bagnoli, who was a venture analyst with Plug and Play when the account was written, and is now an Electric Power & Natural Gas Analyst at McKinsey & Co. “With it, it is possible to leverage deep learning algorithms to train the networks on the devices and shrink their size without the hurdle of sending data to the cloud and, hence, added latency in order to analyze it.”
She also mentioned Pete Warden, noting that he is a TensorFLow Lite Engineering Lead at Google, and has published a book along with Daniel Situnayake entitled, “TinyML: Machine Learning with TensorFlow Lite on Arduino and Ultra-Low-Power Microcontrollers”, which has become a reference in the field.
As of January 2021, the Arduino Nano 33 BLE Sense was the only 32-bit board that supports TensorFlow Lite, making machine learning embedded on hardware accessible to anyone. Arduino collaborates with the startup Edge Impulse to lower power consumption; it supports processing of data at the sensor interface through an inference engine, only sending data when necessary.
“Ultra-low-power embedded devices are invading our world, and with new embedded machine learning frameworks, they will further enable the proliferation of AI-powered IoT devices,” stated Bagnoli.
Read the source articles and information in ZDnet, in a press release from ABI Research, on the blog of Plug and Play, and in the book, “TinyML: Machine Learning with TensorFlow Lite on Arduino and Ultra-Low-Power Microcontrollers.
AI/ML
Trending AI/ML Article Identified & Digested via Granola by Ramsey Elbasheer; a Machine-Driven RSS Bot
via WordPress https://ramseyelbasheer.io/2021/09/30/tinyml-enabling-low-power-inferencing-analytics-at-the-edge/